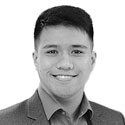
Taxwise Or Otherwise
By Jan Brian P. Despi
FinTech refers to any idea or innovation that improves or optimizes the way individuals or companies conduct financial activities. Early FinTech concentrated on developing add-on products to complement existing financial services.
This combination of finance and technology has spawned a slew of valuable goods and services that redefine financial services and make them more accessible to the general public. Some of these products and services include insurance aggregators, mobile wallets, AI investment management advisers, peer-to-peer (P2P) lending and crowdfunding tools, and platforms for trading financial assets. The cutting-edge solutions that contributed to such technologies include Blockchain, Deep Learning, and Artificial Intelligence (AI). FinTech allows financial services organizations to collect massive amounts of consumer data, determine usage patterns, and even replace human participation with automated algorithms.
The distinction between banks and FinTech is becoming increasingly hazy. It is crucial to understand that “banks” and “FinTech” are not necessarily mutually exclusive. In fact, many well-known banks have evolved from being mere prepaid card providers that link to applications. They have won the right to full-fledged banking licenses after demonstrating to the world that it is feasible to combine sophisticated technology with trustworthy financial services. FinTech can emerge from one of three sources: (1) a stand-alone company develops technologically advanced goods to address unique market concerns; (2) a company develops a full-fledged body to become a complete bank; or (3) a conventional bank incorporates technological advancement by acquiring a smaller FinTech to modernize its service.
SIGNIFICANCE OF ARTIFICIAL INTELLIGENCE IN FINTECH
Artificial intelligence (AI) is gradually gaining a foothold in practically every business in the twenty-first century. FinTech is used mostly to improve and automate different financial operations. With the advent of knowledge engineering, financial institutions employ AI-based models in conjunction with their FinTech apps to maximize operations and revenue.
Some of the major significant uses of AI in FinTech are:
Large-scale wealth and finance management: Traditionally, the wealth management sector has catered to high-net-worth individuals. AI solutions are assisting to considerably expand this industry by allowing it to scale its ability to supply to a much larger segment of the population. In addition to specific financial advice, the AI may analyze spending habits to ensure that customers have adequate emergency money and provide continually updated net worth predictions for improved retirement planning.
Enhanced security: Many FinTech firms and conventional financial institutions are already using AI-based solutions for various fraud monitoring and prevention applications, but there is always room for improvement as fraudsters escalate their attacks.
Contract management: Contracts are an integral aspect of the financial business, as they are in many other sectors. Keeping track of all contracts, whether between institutions and clients or between enterprises, requires a significant amount of effort. AI can assist in speeding up this process by combining optical character recognition (OCR), machine learning (ML), and natural language processing (NLP).
Improved customer services: Through intelligent software bots, AI has been able to fill a need in this field. These bots suggest personalized products and services that better meet customers’ needs and demands. Financial institutions that employ chatbots have ample motivation to keep using and enhancing them, with worldwide savings from chatbot use anticipated to exceed $7 billion by 2023.
RISK FACTORS ASSOCIATED WITH ARTIFICIAL INTELLIGENCE IN FINTECH
While the applications listed above demonstrate how technology is revolutionizing the financial industry, the deployment of AI is not without hazards. The primary factors that need to be understood are:
Embedded bias: The increasing use of AI in the financial sector, which is heavily regulated and where public confidence is critical, has sparked debate over the potential of inherent bias. Embedded bias is defined as computer systems that routinely and unjustly discriminate against some persons or groups of individuals in favor of others. Customer classification algorithms applied in AI/ML might lead to prejudice in the banking industry through price or service quality differentials. Biases in AI/ML judgments are frequently caused by biased training data derived from existing biased processes and data sets, which teach automation models to be prejudicial.
Explainability and complexity: The term ‘explainability’ refers to the concept that AI models and their outputs can be expounded to humans at an acceptable level. The explainability of AI results is critical, especially when utilized in the financial industry. Because they are not easily explainable by the user, AI is sometimes referred to as a “black box.” This trait may make detecting the appropriateness of AI conclusions difficult, exposing businesses to vulnerabilities such as skewed data, inappropriate modeling methodologies, or wrong decision making, thus undermining faith in their robustness.
Privacy factors associated with data: AI raises new and distinct privacy concerns. Big data privacy problems are well recognized and even precede the mainstreaming of automation. Tools have been created to aid in the preservation of data anonymity and data subjects’ privacy. Legal data policy frameworks are being implemented across the world to address these problems. However, the resilience of AI models in limiting data leakage from the training data set poses additional privacy problems.
Cognizant of the abounding risks, enterprises should leverage on the agile nature of technology and adapt to new work methods. This would reshape the organizations’ ways of doing things through the use of intelligent software bots, minimize manpower costs by utilizing AI and robotic process automation, and most importantly, enhance security, employee engagement, and client satisfaction. As the financial sector’s use of AI and ML continues to surge, it is becoming a must to have professionals who are very much capable of optimizing the usage of advances in processing power, data storage capacity, big data, and modeling.
The views or opinions expressed in this article are solely those of the author and do not necessarily represent those of Isla Lipana & Co. The content is for general information purposes only, and should not be used as a substitute for specific advice.
Jan Brian P. Despi is a senior associate at the DTS Department of Isla Lipana & Co., the Philippine member firm of the PwC network.